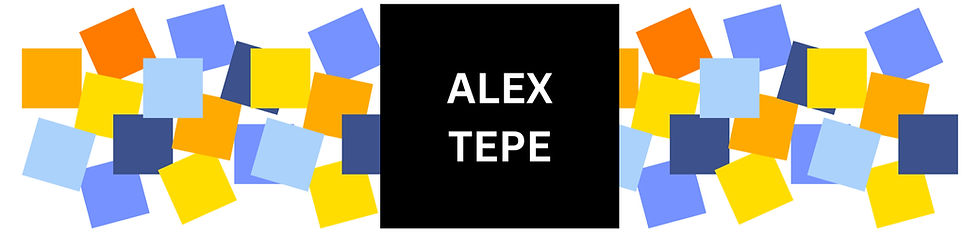
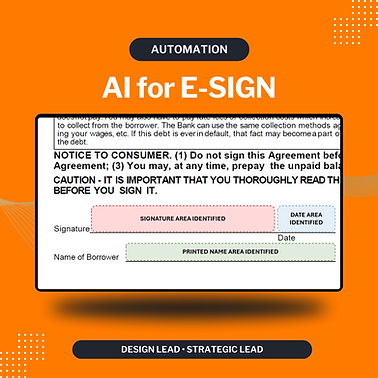.png)
Ai for E-Sign | TL;DR
I led the design and strategy for an Ai-based feature that would automatically "tag" E-Sign documents to reduce manual effort from users, and reduce barriers to automation for smaller customers.
I co-created an MVP solution with a leading Auto Finance lender to provide large datasets and to consult on design and priority of features.
​
With the assistance of OCR and Computer-Vision, the model was able to recognize and apply the correct tags with 86% accuracy when encountering a new document.
The Challenge
In E-Signature, the act of "tagging" documents (defining areas to sign, date, etc.) is by far the most time-consuming task for users. While this action can be automated through API - this requires a large investment from the owner of the document library, must be continuously updated as the documents evolve, and not all customers are willing/able to invest in that automation.
The Theory
For financial institutions who rely on manual document tagging for E-Sign, Auto-Tagging could be a faster and more affordable option compared to technical integration or continued reliance on manual tagging. Unlike the alternatives, Auto-Tagging would be turnkey, flexible, and would bring operational efficiencies to financial institutions.
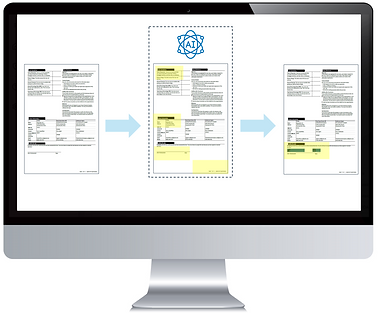

The Results
Through collaboration and prototyping with data-science engineers, we found that the model could, indeed, effectively recognize tag locations on documents if given sufficient training data.
Auto-Tagging allowed us to:
-
Recognize and apply the correct tags with 86% accuracy when encountering a new document
-
Recognize and apply the correct tags with near 100% accuracy for trained-set documents
-
Create a new SKU and increase E-Sign per-package revenue by 33% ​